Around 600 people gathered at this year’s Swiss Conference on Data Science (SDS) to discuss relevant use cases and research initiatives in the field of data science. Star guest Leandro von Werra from Hugging Face ushered in the comeback of open AI models. As scientific partner of the conference, researchers from the ZHAW Datalab contributed with talks and workshops – and won the best paper award.
It is a rainy day at the Circle – the Convention Center at the Zurich airport. The venue is buzzing with the chatter of data scientists, industry leaders and AI researchers from Switzerland and beyond. The SDS manages to connect the world of research and application, by bringing together speakers from industry, academia and politics. The 2024 conference tackled current data science topics, such as AI, cybersecurity, visualisations and more.
Renaissance of Open Models
From start to the end of the conference day, the main hall is fully packed. No one wants to miss the keynote by Leandro von Werra, “Chief Loss Officer” at Hugging Face. First, he gives an overview of the ingredients that are needed to cook up a Large Language Model (LLM): the model (transformer), data (the most important ingredient) and compute (lots of GPUs). Now the question: How can the recipe be improved? Remarkably, the scaling laws apply: by scaling up, and including more data, the model improves. Von Werra does not think that we have reached the end of scaling up – there is more data out there, that can refine models, for example data from sources other than text. Another way to get a good model without scaling up is to train it longer, which saves money in the process. Today, even though closed models from big companies perform best, there is much potential and advantages in developing and training open models. Firstly, the data used is transparent and biases can be detected. Secondly, “the performance of open models is catching up to closed models,” says von Werra. “When using high quality data sets and fine-tuning the model, it can surpass GPT4 performance if it is trained right”, he adds.
Evaluating AI-Generated Text
Like Leandro von Werra, ZHAW researcher Mark Cieliebak from the Centre of Artificial Intelligence talks about “good” AI models. Unlike von Werra, however, he focuses not on the quality of the input – the ingredients – but on the quality of the output of generative models. The dish to be analysed is the generated text. “Comparing Large Language Models with each other is like watching racing cars – they evolve and improve so quickly”, says Mark. When evaluating text generation by AI, the first thing to consider is what constitutes “good text”. Best practice is to analyse consistency, relevance, fluency and coherence. These four measures can be evaluated by humans or automatically. Both methods have their shortcomings, such as human annotation reproducibility or AI hallucinations. Cieliebak and his team have developed solutions for these hurdles within an SNF-funded project, and they are currently looking for industrial partners who want to evaluate “their” generative systems.
Ensuring Fair and Clean Data
Later in the day, in a very crowded room, ZHAW researcher Lilach Goren Huber explains how AI can detect anomalies in data samples. Since errors in the data can lead to an AI model with foul output, new methods are needed to “clean the dirty data”. Huber presents an unsupervised method that uses an intermediate step to remove anomalies in data samples and that can be used with any model. In another talk, Christoph Heitz delves into the philosophical notion of group fairness and how it can be applied to the impact assessment of algorithms. Unfairness is defined as a treatment that systematically imposes a disadvantage on one social group relative to others. Group fairness can then be formalized through the principle of equal expected utility. As prediction-based decision systems impact the lives of many people, it is absolutely essential to include fairness in the algorithm design.
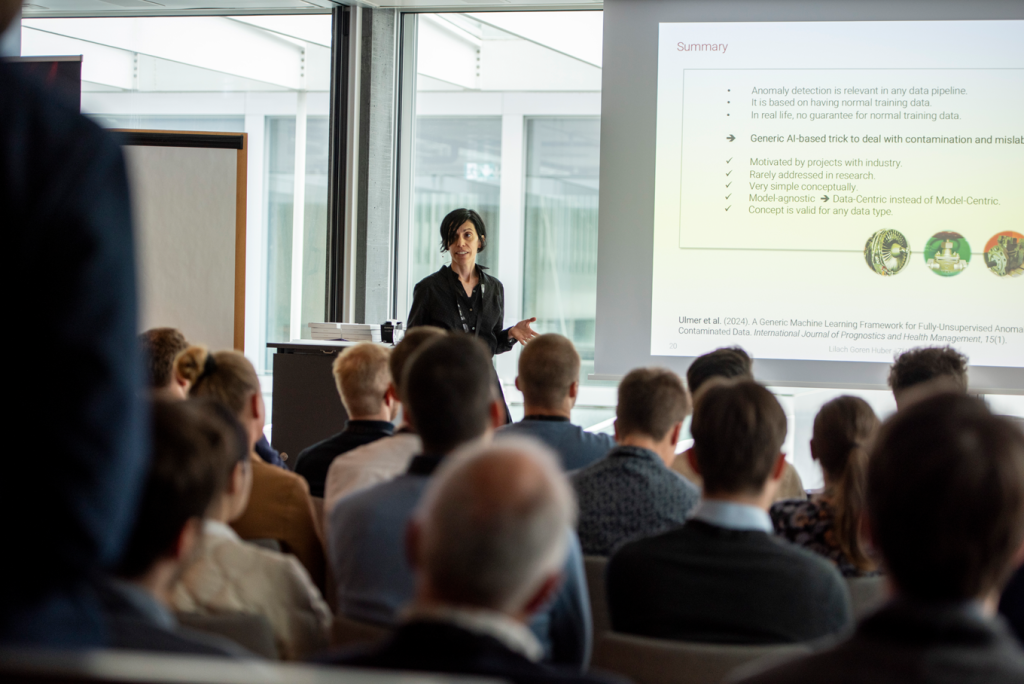
The Power of Visualizations
In the second keynote of the day, Daniel Keim from the University of Konstanz talks about the power of visual analytics. “Computers and humans together are very powerful”, says Keim. By visualising data, complex knowledge can be grasped more easily, for example in analysing financial data, network security or sports data. Imagine you can see on a digital map the spread of a computer virus and detect intrusions with AI. This can help the control room of an organisation. Right now, such a visual dashboard is implemented at the University of Konstanz. “What about visualisations in Virtual Reality (VR)?”, asks someone from the audience. Visualisations in VR are not there yet, but technology improves quickly and it’s around the corner, answers Keim. So far, he and his team used 3D VR models only in investigations of complex crime scenes, but there is potential for more use cases.
Addressing Climate, Health, and More
Researchers at the conference touch upon many more applications of data science to solve pressing issues, for example about AI as a tool for mitigating climate change. Thomas Brunschwiler (Research Manager at IBM) presents the results from a whitepaper study with more than 50 collaborators and examples of AI applications such as in flood risk estimation. The study provides recommendations for decision makers and data scientists and the team wins at the end of the day the AI for Social Impact award. In a poster presentation, a team from India showcases their work with a robotic arm that supports patients after a stroke. It uses a predictive model which forecasts the movements of the patients, supporting them only when needed. The scientific community at the conference uses big data and AI for good and is happy to discuss use cases with industry partners during breaks. After the conference, ZHAW researcher Philipp Denzel says that one of his highlights was “the exciting discussions during the breaks with like-minded people I met for the first time and with old friends I met again.” Another highlight Philipp Denzel points out, is that he and his co-authors won the Best Scientific full Paper for their work “Towards the Certification of AI-based Systems”.
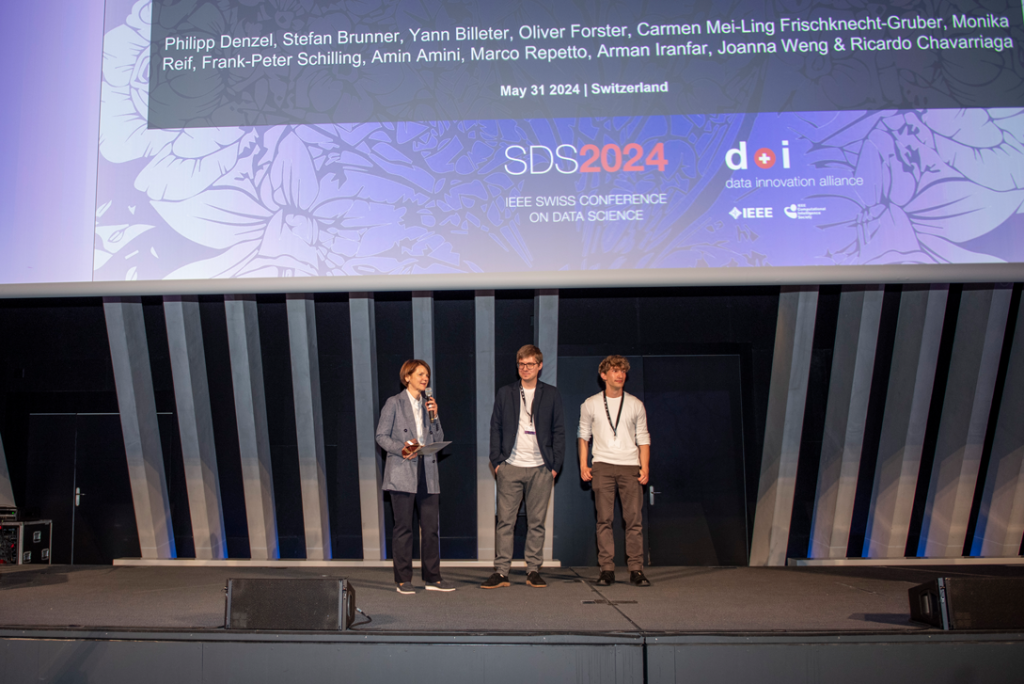
The conference is a success for ZHAW researchers, participants from industry and partners and the organisation team. Manuel Dömer, co-head of the ZHAW Datalab, summarises: “I was amazed by the diversity of applications for data science methods in industry and academia that we saw in the various talks. It clearly shows how we, as a community, manage to create value from data in the various fields thanks to recent advances in AI combined with effective data engineering and established statistical methods.” The day ends with celebrations of the award winners, networking and an apèro.
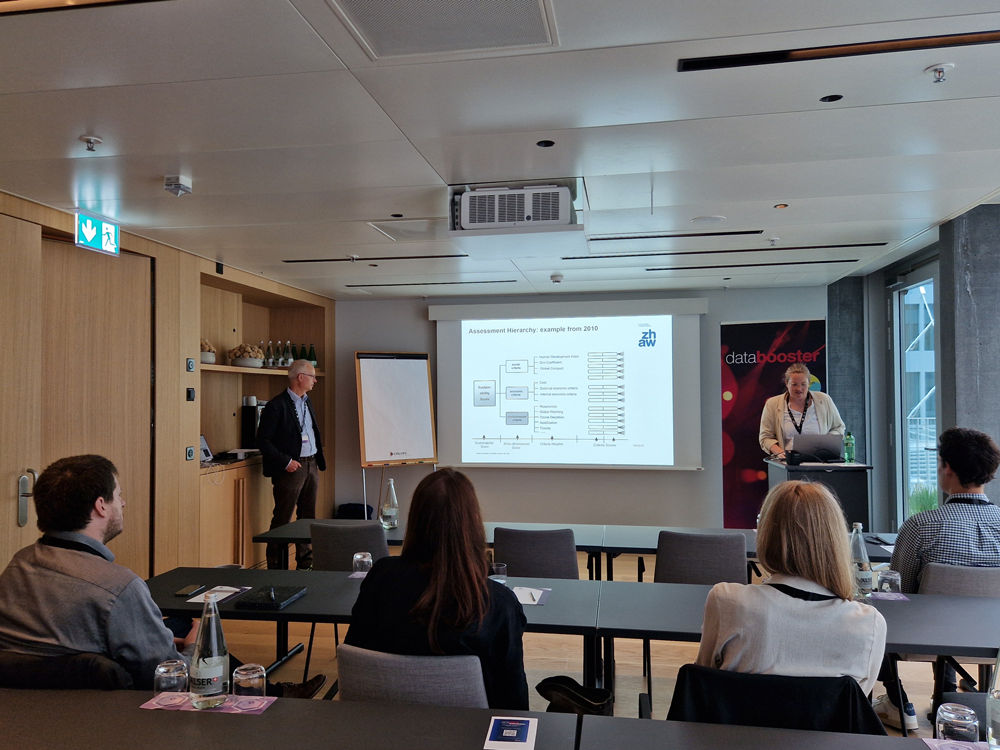
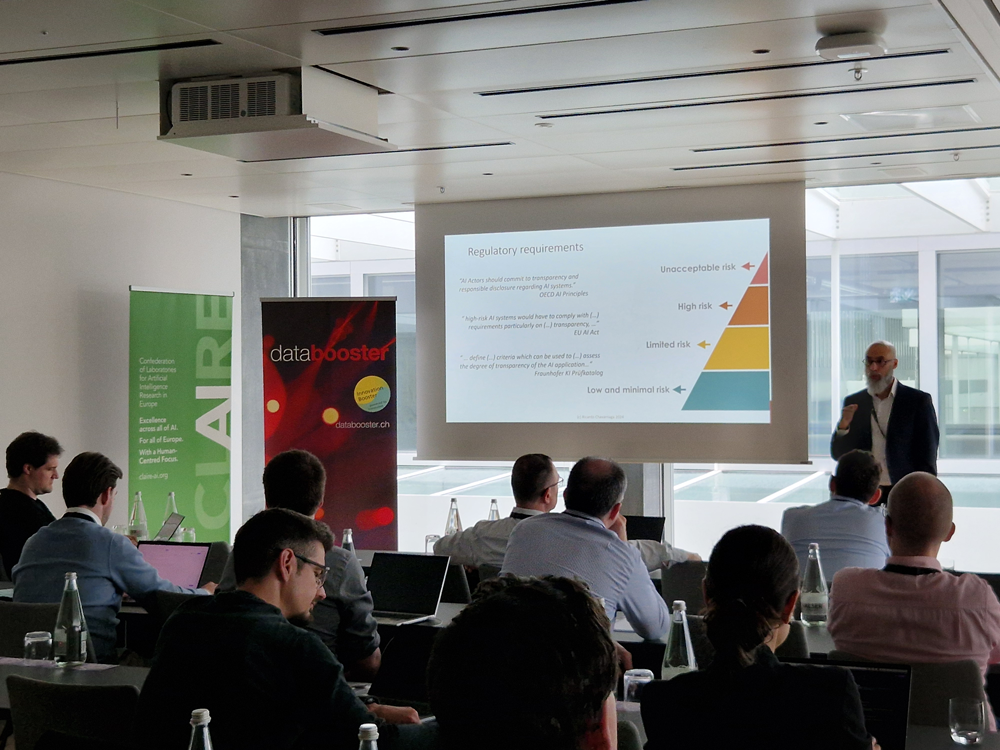
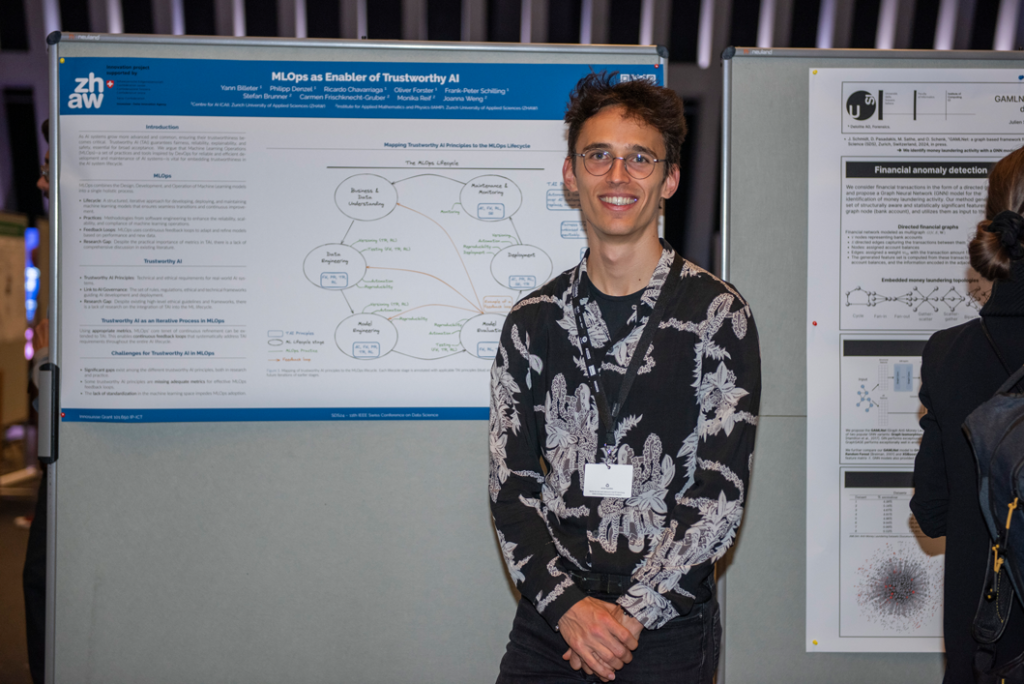
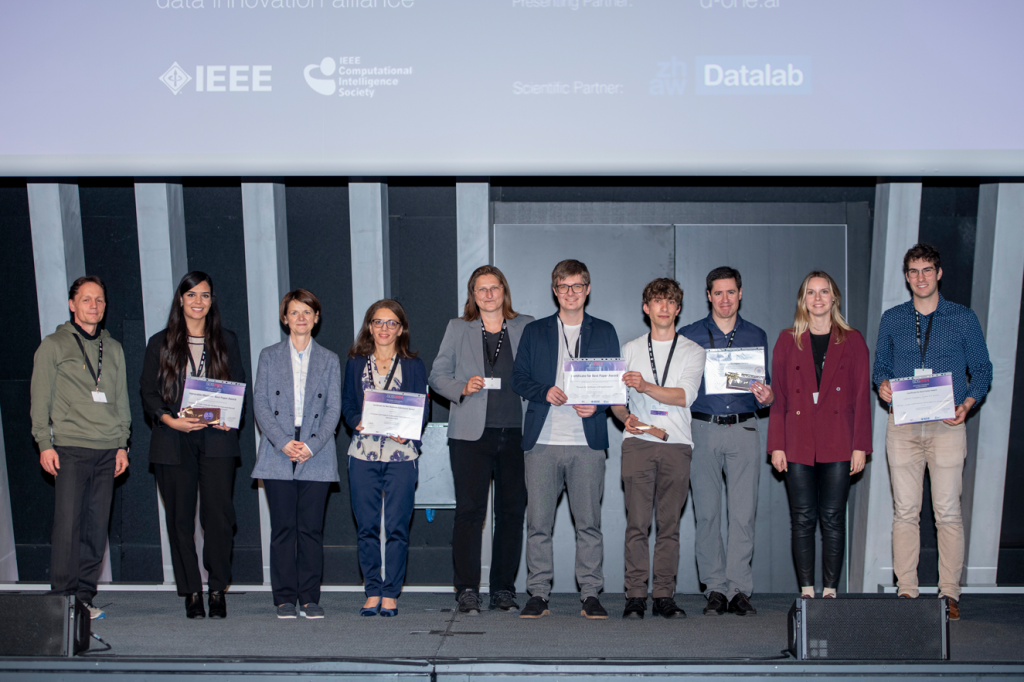
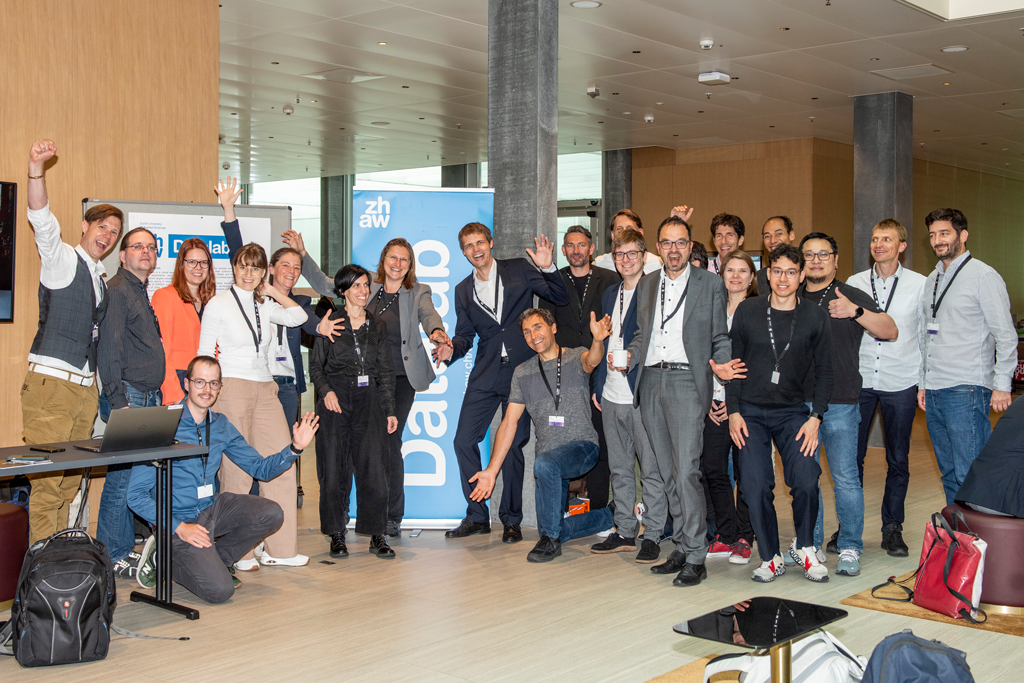
Photo credit goes to Simone Frischknecht (www.simonefrischknecht.ch).
What is the ZHAW Datalab? The Datalab was founded in 2013 as one of the first Data Science labs in Europe. It currently comprises 12 institutes and centers from 4 different departments (School of Engineering, Life Sciences and Facility Management, School of Management and Law and Applied Linguistics). The members have a background in research and teaching, established a network of data scientists and have connections to partners from industry. The research agenda covers areas such as database and big data technology, data mining, statistics and predictive modelling, machine learning, privacy, security and ethics and much more. |
More information: