343 hours of spoken Swiss dialect, one AI model, many questions. How different are Swiss dialects from each other? And can they be translated from Swiss German to Standard German with AI? “Yes, we were surprised how well that worked” says Jan Deriu from the Centre for Artificial Intelligence at ZHAW.
Switzerland has a rich diversity of different dialects, and they are considered important markers of regional identity. In German speaking regions, Swiss German dialects are spoken in everyday situations – formal and informal ones and written mostly in informal contexts. A recent SNF project divided the Swiss German landscape into seven dialect regions: Basel, Berne, Central Switzerland, Eastern Switzerland, Grisons, Valais, and Zurich. Researchers from ZHAW and FHNW compiled a large corpus of these dialects and trained an AI model on it that translates Swiss German speech into Standard German text. The project provided insights into the challenges of building Swiss German speech translation systems, specifically focusing on the impact of dialect diversity and differences between Swiss German and Standard German. The paper of the project team was accepted at the Findings of the Empirical Methods in Natural Language Processing (EMNLP 2023) in Singapore. Jan Deriu, researcher at the Centre for Artificial Intelligence, points out the key insights.
Jan, what exactly did you want to find out in this project?
We wanted to establish how dialects interact with each other and what consequences this has for training AI models. For example, when we train an AI model with Bündnerdeutsch, the Swiss German dialects spoken in the Grisons, can it also translate Zurich German? In other words, can we see the linguistic distance of the dialects also in the data, in the output of AI models? Another aspect we were interested in was the translation of spoken Swiss dialect into Standard German text. How well does this work, considering how different Swiss German is from Standard German? And Swiss German is very different: we do not use the preterite tense, the grammar and phonology differ, and we use many lexical items that are distinct from Standard German. In our research, we mainly looked at the past tense and vocabulary. Can an AI recognize that Swiss German “Guetzli” is the same as Standard German “Keks” or “cookie”?
And what did you find out? Does the data match the linguistic prediction?
Yes, we were surprised to see how well it matched. Walliser German is the most linguistically distant dialect from other dialect regions. And sure enough, systems that are trained only on Walliser German perform badly on other dialects, and systems not trained on Walliser German will not perform well on this dialect. Also, the dialects from the Grisons are quite linguistically distant and the output showed this. Dialects that are quite “central”, i.e. similar to many other dialects (such as Zurich German) positively affect others when included in the training data. So, when training a model with a central dialect, Zurich German, only, Central and Eastern Switzerland dialects can be translated almost perfectly (90% score). When we trained the model with all the data but left one dialect out, the largest overall drop in performance occurred when we left out Zurich German or Basel German.
Was the AI model able to translate “Guetzli” to “Keks”?
That proved to be difficult. In the second part of our study, we found that the usage of preterite and vocabulary specific to Swiss German impacts the quality of the Standard German output negatively. When we looked at the Standard German transcript (output of the model that was trained on all Swiss German dialects) and the Standard German reference we found a mismatch. In the future, we want to find methods that handle these differences.
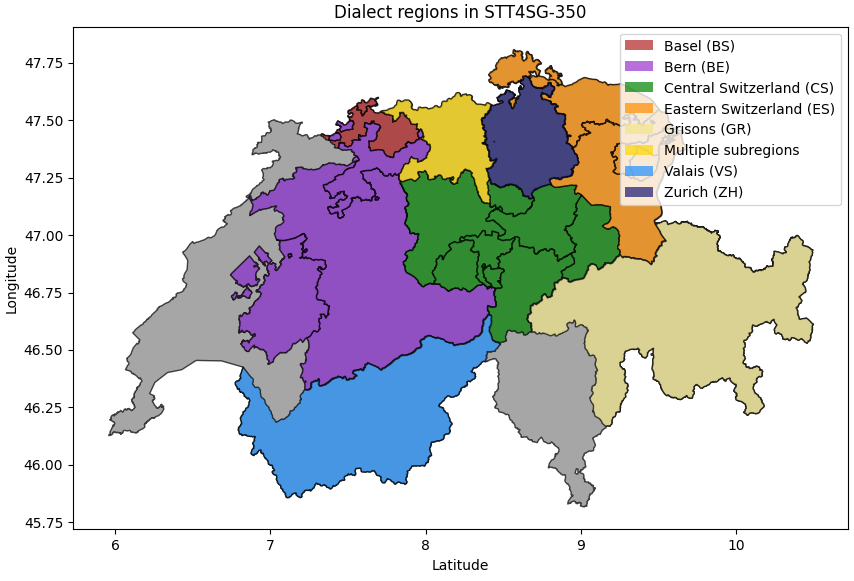
Where does the data come from? Are the 343h of spoken dialect part of your Citizen Science project “Swiss dialect collection”?
It is not part of our previous project, the Swiss dialect collection, but we used the same tool and similar methods. The reason we collected the data ourselves was that we wanted to have an even distribution across gender, age and dialect region. Therefore, we asked 30 people per dialect region to record texts – not an easy task for regions with few speakers. Our corpus now consists of 343 hours of speech translation data. That is, it contains audio in Swiss German and the corresponding Standard German sentence. Our corpus also contains a test set with 5 hours of audio for all 7 dialect regions that is based on the same set of sentences. This allows for conclusive comparisons.
You used this data to train a model. Did you train it from scratch?
Nowadays the practice is to use pre-trained foundation models, however, we also tested our hypotheses on a randomly initialized model. Thus, we used three different models, two that had been pretrained on many different languages, and one randomly initialized. We then fine-tuned/ trained the models with our data and now have a good Swiss-to-Standard German speech translation system.
Can others use your corpus or the AI model?
Yes, it can be accessed for research or commercial use. You need a licence for both use cases, but the license for researchers is free. If anyone is interested, feel free to get in touch with us.
What happens now with the model and what else are you and the team working on?
Theoretically, the AI model can be used to translate spoken dialects to written Standard German and we will coordinate this with the FHNW. There are two demos available: one for short live recordings, and one for long audio files. We have also applied for a new project in which we want to build a text-to-speech model for Swiss German, so the other way round. A project I am currently working on is the HAMiSoN project, where we are analysing together with European partners how misinformation is spread through YouTube. In another project called ChaLL, Manuela Hürlimann is working on a chatbot that helps students learn English. We have a lot of interesting projects going on and are also continuing to investigate how well chatbots perform.