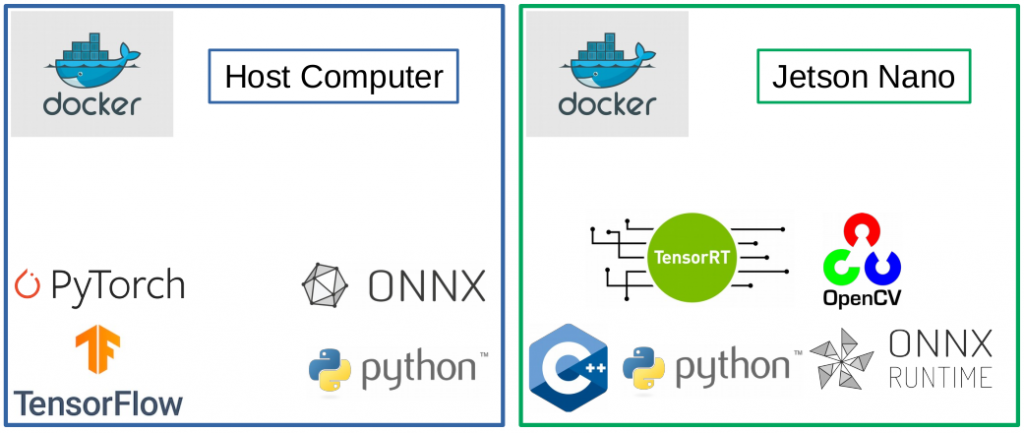
Running Artificial Intelligence Algorithms Directly on Jetson Nano and Microcontrollers
Running artificial intelligence (AI) algorithms, such as neural networks, directly on embedded devices has many advantages compared to running them in the cloud: One can save significant amounts of cloud storage, reduce power consumption and enable real-time applications. In addition, privacy is increased and required bandwidth reduced because only the AI algorithms results are forwarded […]