“The major winners will be financial services companies that embrace technology.”
Alexander Peh, PayPal and Braintree
Over the last few years, Reinforcement Learning (RL) has gained significant attention as a framework for learning optimal decisions even in complex environments. Moreover, RL is currently considered one of the most promising research areas in machine learning and has already demonstrated immense potential for solving sophisticated real-world problems, such as in robotics, resource management or health care.
It is expected that RL algorithms have the potential to disrupt the financial service industry significantly and revolutionize the development of new financial market products.
However, despite all major academic breakthroughs a broader deployment of RL in financial applications is currently lacking, and practical implementations mostly remain hidden as intellectual property of only the largest global players. One fundamental reason for this is the significant hurdles that practitioners are still facing in building such models in real-world settings.
The Innosuisse Innovation project “Strengthening Swiss Financial SMEs through Applicable Reinforcement Learning” addressed these hurdles by identifying outstanding practical challenges when applying RL and building exemplary and openly accessible use cases that showcase the vast potential of RL applications in finance.
Overall, the project team evaluated four use cases (Optimal Execution via RL, Factor Rotation, Recommender Systems, and Market Simulation), of which two were documented and deferred and two were successfully implemented: Optimal Execution via RL and Factor Rotation. All results of the implantation are freely accessible via a fully documented Git repository and are accompanied by research output. The relevance of the use cases as well as their implementation was validated throughout the project in close collaboration with the Swiss financial industry.
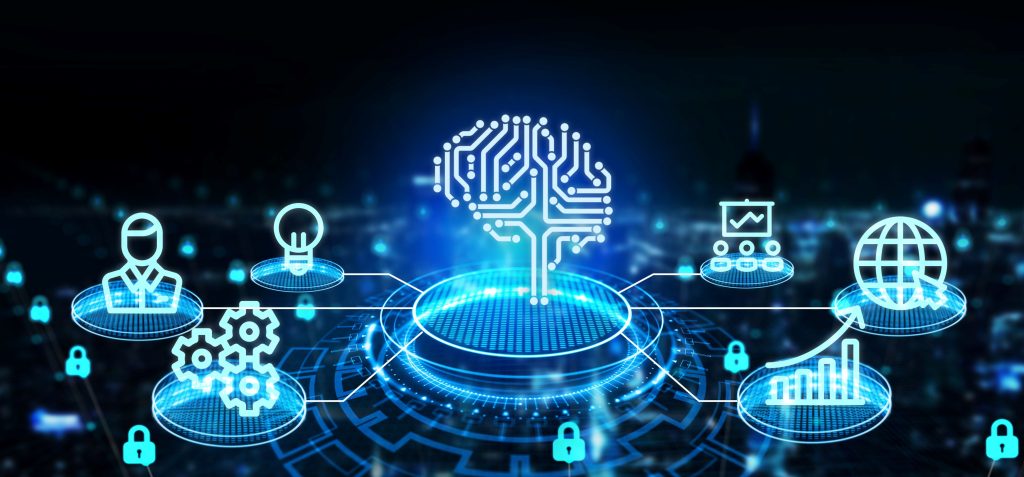