In this article, the authors construct a pipeline to benchmark hierarchical risk parity (HRP) relative to equal risk contribution (ERC) as examples of diversification strategies allocating to liquid multi-asset futures markets with dynamic leverage (volatility target). The authors use interpretable machine learning concepts (explainable AI) to compare the robustness of the strategies and to back out implicit rules for decision-making. The empirical dataset consists of 17 equity index, government bond, and commodity futures markets across 20 years. The two strategies are back tested for the empirical dataset and for about 100,000 bootstrapped datasets. XGBoost is used to regress the Calmar ratio spread between the two strategies against features of the bootstrapped datasets. Compared to ERC, HRP shows higher Calmar ratios and better matches the volatility target. Using Shapley values, the Calmar ratio spread can be attributed especially to univariate drawdown measures of the asset classes.
Jaeger, Markus; Krügel, Stephan; Marinelli, Dimitri; Papenbrock, Jochen; Schwendner, Peter, 2021. The Journal of Financial Data Science 3(3), pp. 31-51.
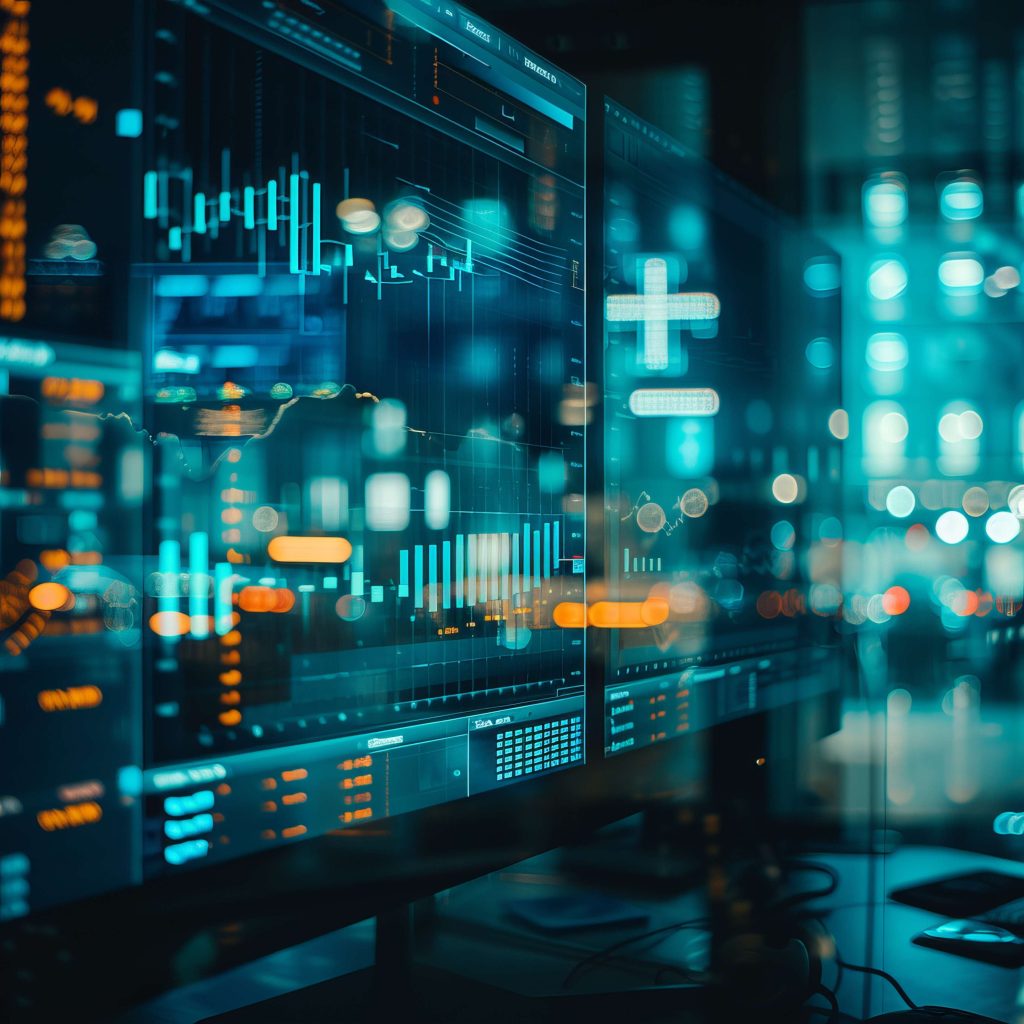